Modern computer vision systems are remarkably effective at solving specific tasks in specific visual domains. These systems work because they are trained on huge curated datasets tailored to the task they aim to solve. The cost is that we need a different vision system for each new task: a system that analyzes MRI images cannot be instead used to recognize pedestrians, unless it is extensively retrained. My goal, in contrast, is vision systems that work regardless of the domain in which they are deployed. I believe the way to achieve this is to develop methods that better relate knowledge in one domain to prior experience in another, and I will study three ways of doing so: 1) learning domain-general perceptual representations, 2) learning to translate between domains, and 3) developing representations primed for adaptation. Success would take us a step closer to general-purpose artificial visual intelligence.
Fellow
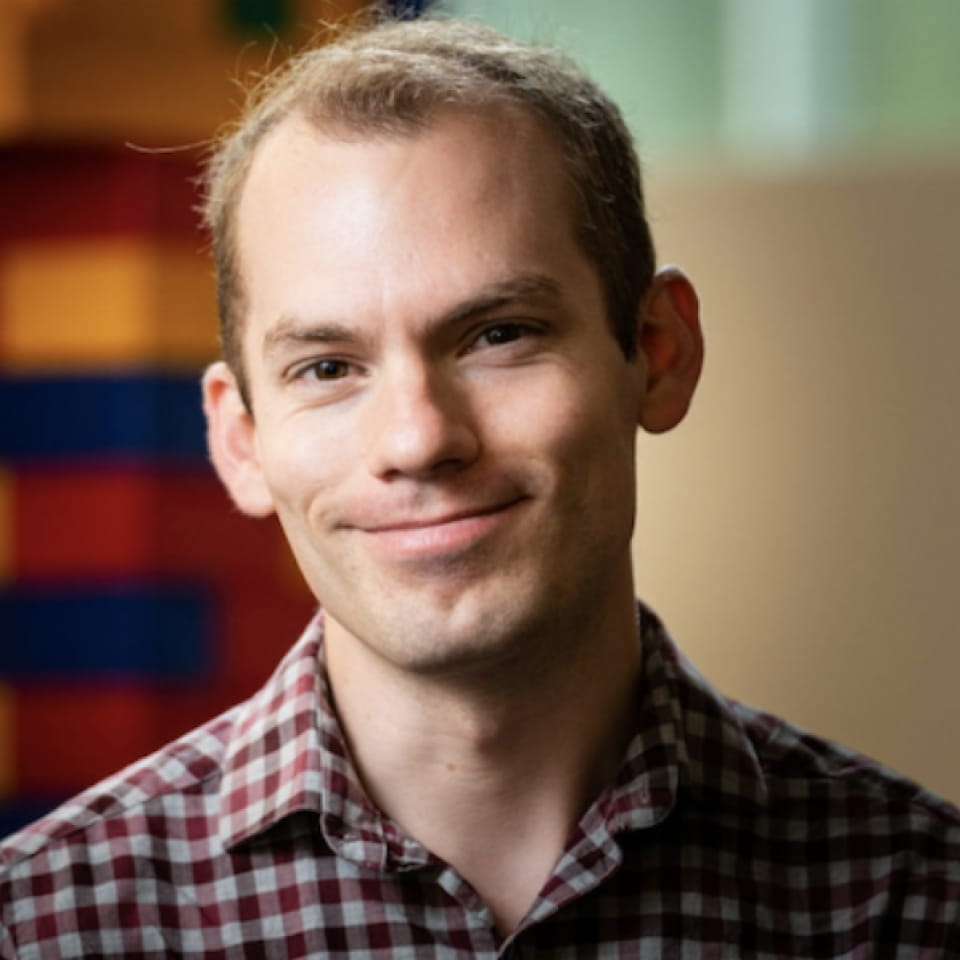